Investing in enterprise-grade Language Models (LLMs) promises great returns, but only if companies can avoid common missteps. By recognizing these pitfalls and implementing effective strategies, businesses can maximize the potential of LLMs.
Understanding the LLM Landscape
Every ambitious company today is vying for a technological edge, keenly eyeing advancements like enterprise-grade Language Models (LLMs) that promise efficiency and innovation. However, the journey to leveraging these marvels is fraught with common missteps. Let's unpack some of these pitfalls and chart a roadmap to successful LLM adoption.
Every company aims for efficiency, growth, and innovation. But in the race to adopt the latest technologies, there are pitfalls. One such technological marvel is the enterprise-grade Language Model (LLM). Here's a dive into the common mistakes companies make when investing in these LLMs and how to avoid them.
1. Mistaking Tools for Commitment
Just investing in an enterprise-grade LLM doesn't guarantee effective use. The hurdle isn't the absence of a tool - it's our reluctance towards change and the discomfort of adopting new practices.
The Solution is to:
- Focus on training. Ensure your team knows not just how to use the LLM, but wants to use it. Addressing the human element is key - tools are only as effective as the people using them.
- Address the human element. Tools are only as effective as the people using them. Making behavioural and mindset changes across the organization is crucial for success.
2. Relying on the "Cutting-Edge" Factor
Companies often adopt LLMs to enhance data communication. The initial advantage is temporary - competitors will soon catch up, levelling the playing field.
The Solution is:
- Prioritize behavioural and mindset changes in your organization over adopting the latest tech. LLMs have the potential to enhance nearly all functions when used right, significantly boosting work quality and speed.
- Realize the sustained advantage comes from how LLMs are integrated, not just owning the cutting-edge tech. Focus on the ripple effect on roles, recruitment, and structure.
3. Mixing High-Tech with Outdated Infrastructure
You don't want to integrate an advanced LLM into an outdated organizational structure - it's going to be a costly and resource intensive mistake. The systems, task prioritization, and delegation methods might not be optimized to harness the LLM's full potential.
The Solution is:
- Have a comprehensive integration plan before investing. Understand the ripple effect on operations.
- Reassess organizational roles, recruitment strategies, and structure to optimize for the LLM. The tech is only one piece - effective adoption requires evolution.
4. Not Hiring the Right Talent
LLMs require specialized expertise to implement and manage properly. Without the right talent, companies can struggle to use LLMs effectively.
The Situation: You've invested in a powerful enterprise-grade LLM, but don't have the experts on staff to optimize it. The technical nuances of prompt engineering and model training are new skills your team lacks. There's no leadership focusing on an AI adoption strategy.
The Solution:
- Hire prompt engineers to craft effective prompts and fine-tune model training. This expertise makes or breaks LLM success.
- Appoint a Chief AI Officer to lead strategy. Having leadership dedicated to AI adoption is crucial for integration and realizing ROI.
- Invest in developing in-house talent. Arm your team with the skills needed to leverage LLMs through training programs and learning resources.
Getting the most from an enterprise-grade LLM requires specialized skills. Building a team with prompt engineering and AI leadership expertise paves the way for maximizing value.
The key takeaway? The sustained competitive advantage from enterprise-grade Generative AI/LLMs comes not from simply owning the tech, but from holistically evolving people, processes, and infrastructure to unlock its full potential. With the right adoption strategy, companies can leverage LLMs to drive transformative efficiency, growth and innovation
Deploying Generative AI in the Right Way
The potential of generative AI is clear. But how can companies actually integrate these powerful models into their operations? Successfully deploying generative AI requires three key elements:
1. Strategic Process
First, organizations need a thoughtful process and plan for deploying AI. Which business processes should be augmented first? How will workflows need to adapt? What risks or challenges may arise? By proactively addressing these questions, companies can ensure a smooth and effective integration.
The process should involve stakeholders at all levels to assess needs, surface concerns, and gain buy-in. Ongoing iteration and testing are also crucial to refine the AI deployment over time.
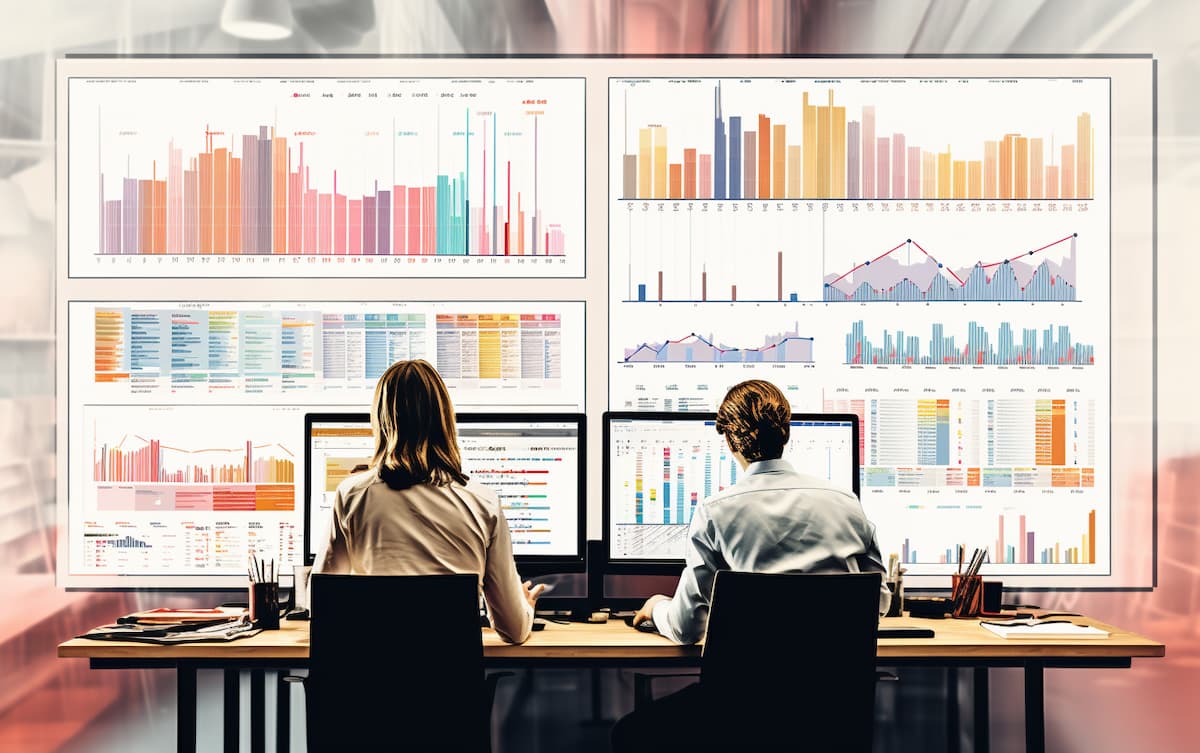
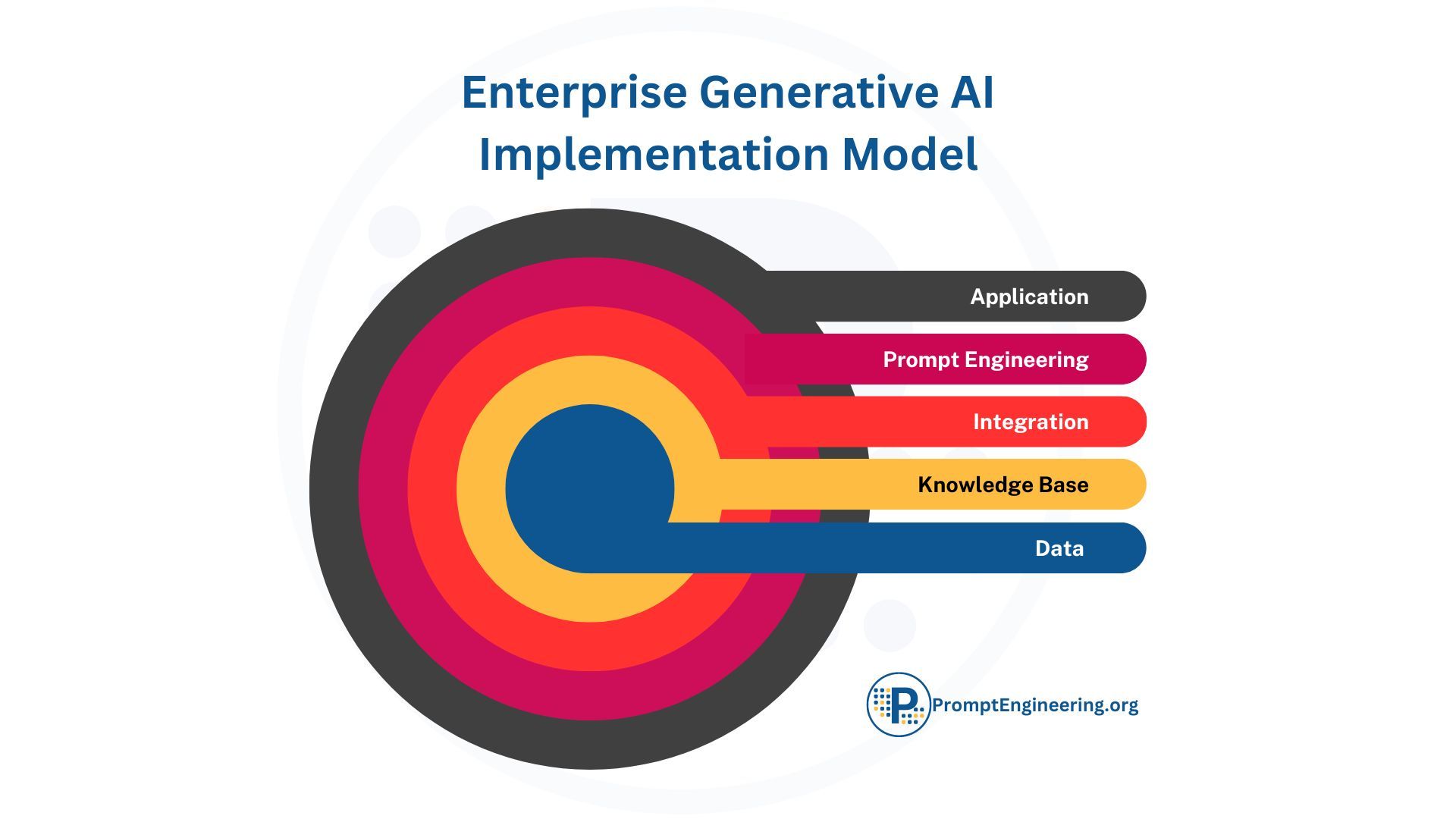
2. Tailored Technology
Next, companies must select the right AI technology for their specific use cases. The space is evolving rapidly. There are cutting-edge models like GPT-3 and DALL-E along with more specialized tools for distinct tasks. Organizations should fully evaluate their options to determine the best tech for their needs.
Key criteria include accuracy, efficiency gains, ease of integration, and total cost of ownership. Assessing tradeoffs around build vs buy decisions will help strike the optimal balance.
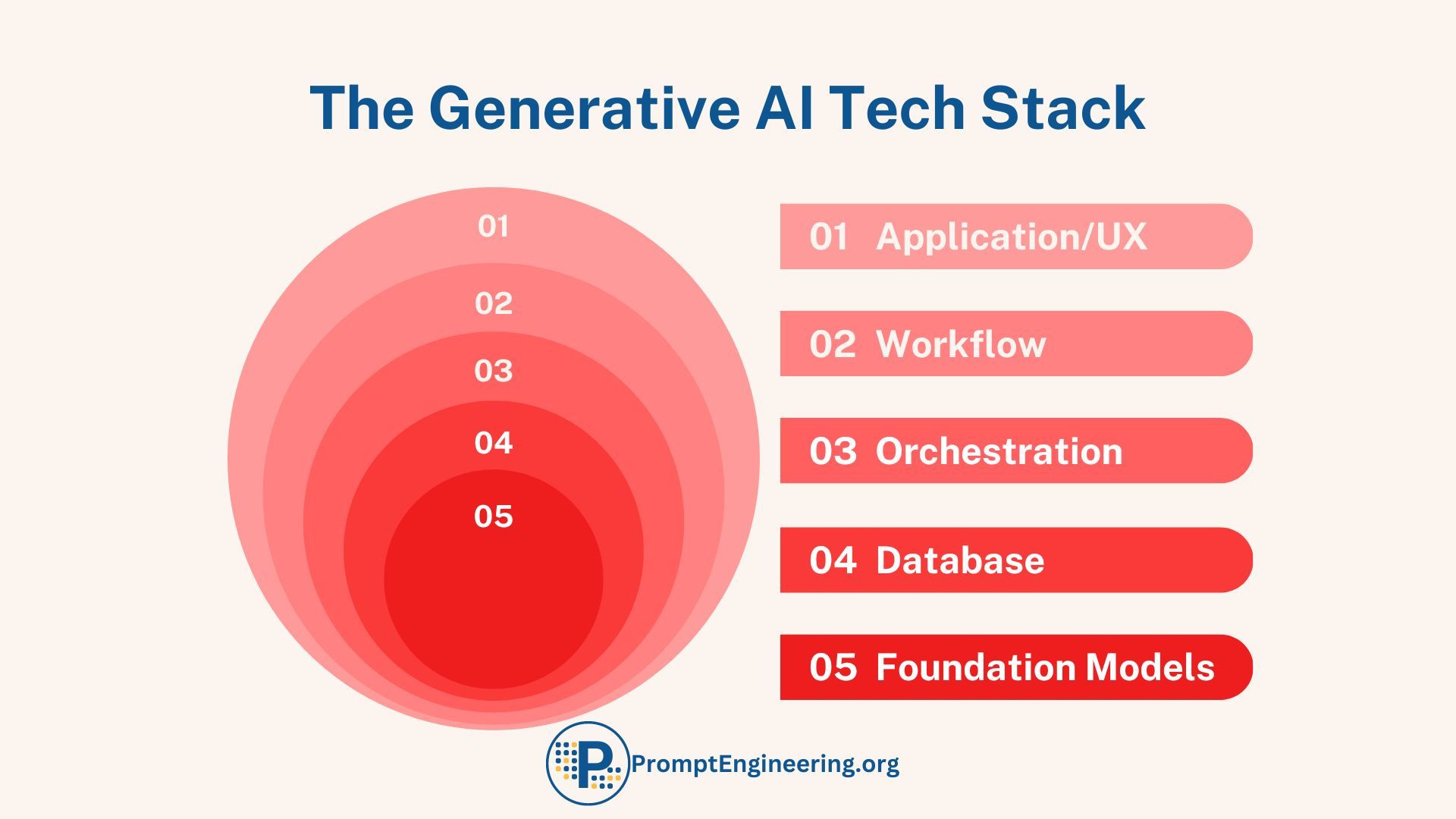
3. Enabling Roles
Finally, companies need the right mix of human roles and responsibilities to enable generative AI. A new capability like "prompt engineering" is required to translate business problems into effective AI prompts. Domain experts ensure output quality. IT specialists can handle technical integration.
Dedicated roles to monitor model performance, suggest improvements, and communicate impact are also important. As technology evolves, so must the surrounding organizational structures and skill sets.
By taking a holistic approach across process, technology, and people, companies can pave the way for successfully unlocking the power of generative AI.

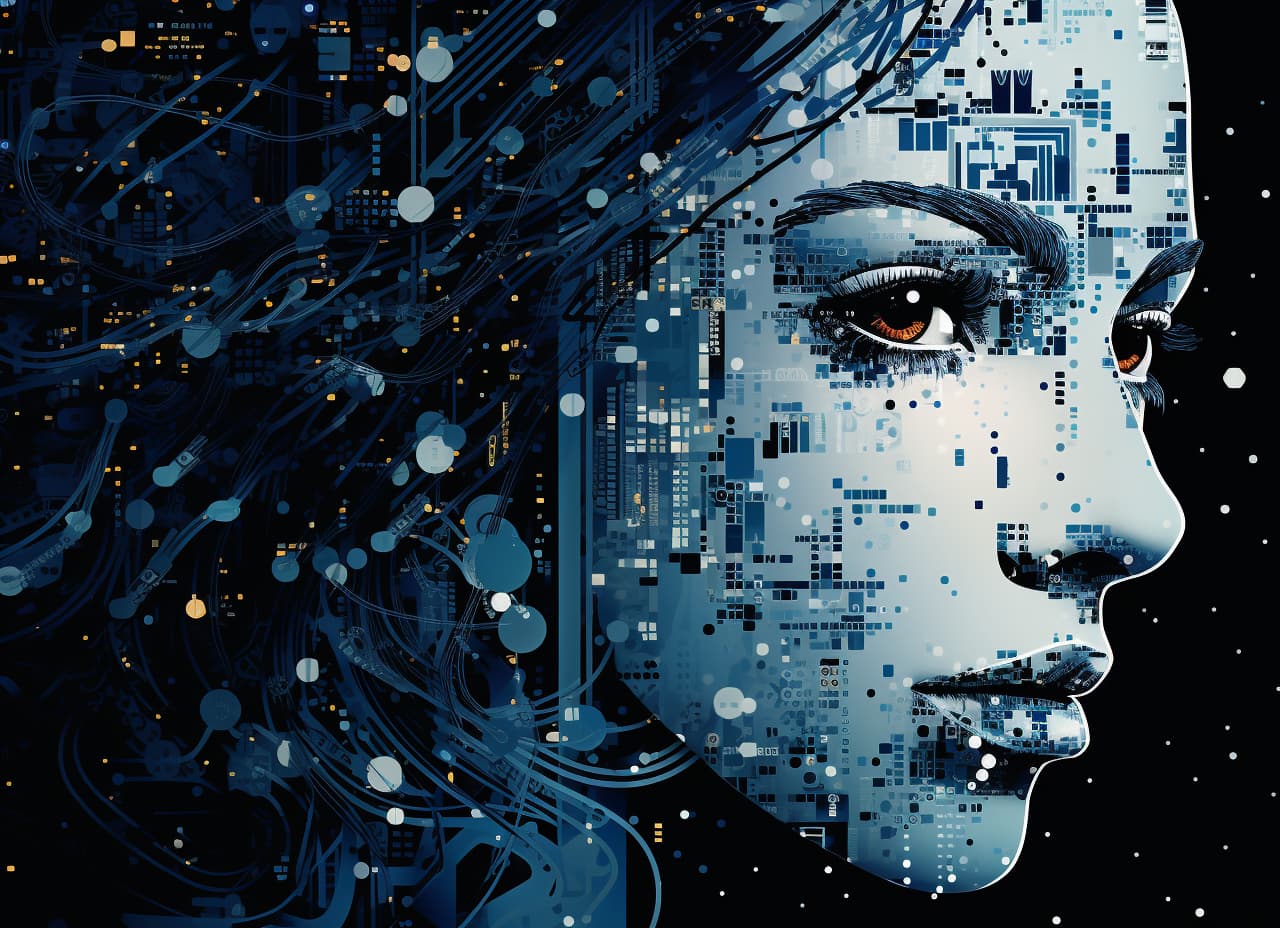