Artificial intelligence (AI) promises immense opportunities for organizations to automate processes, gain insights, and enhance productivity. However, successfully integrating AI across an enterprise is a complex undertaking requiring careful planning and phased execution. This article provides a comprehensive guide to strategically implementing and scaling AI solutions based on leading practices. It outlines a practical playbook for organizations to follow when launching AI pilots and expanding usage company-wide in a measured, responsible manner.
This is a follow-up to the previous article in the series. Please review our Strategic Framework for Enterprise Adoption of Generative AI and The Generative AI Stack
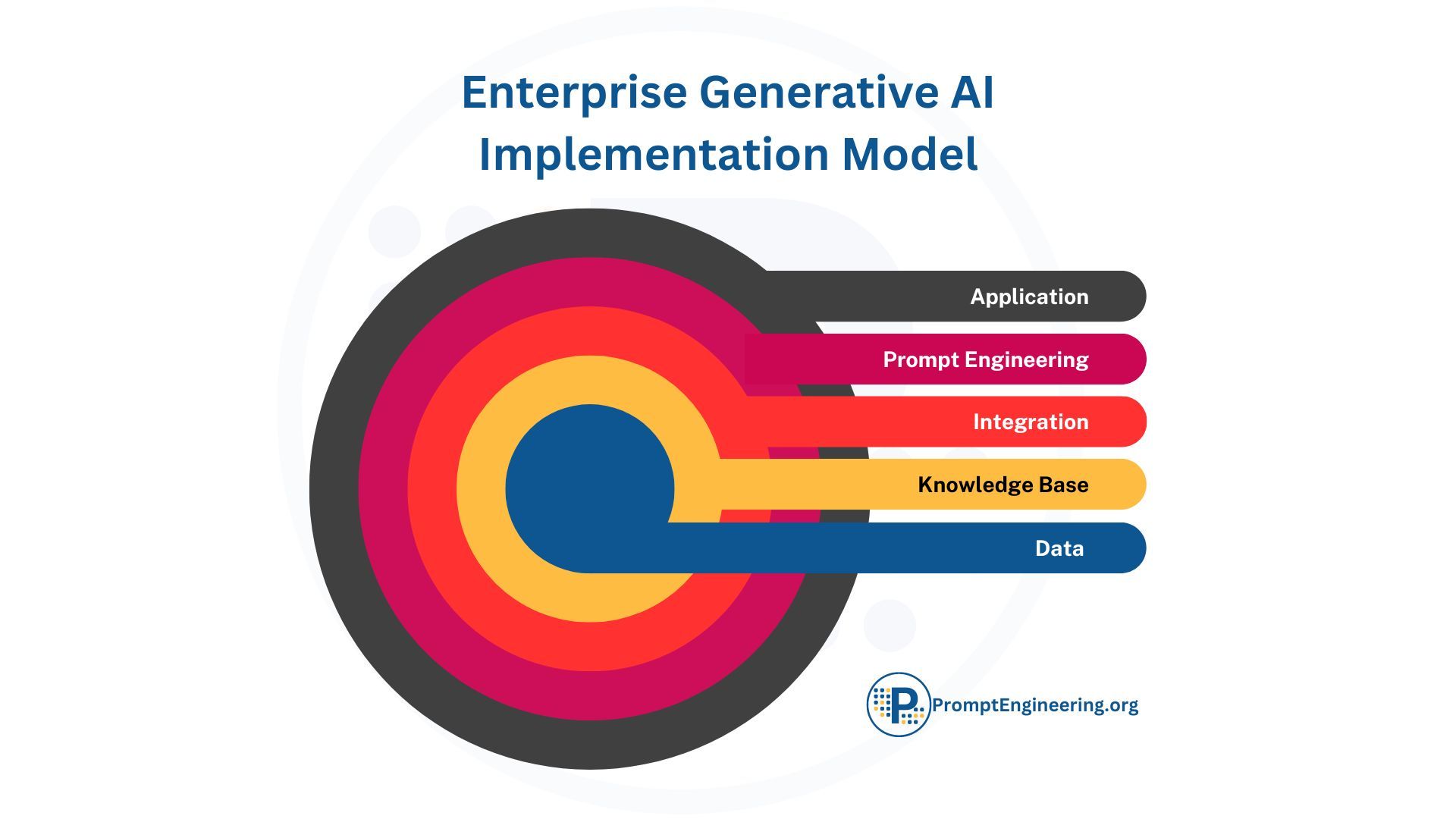
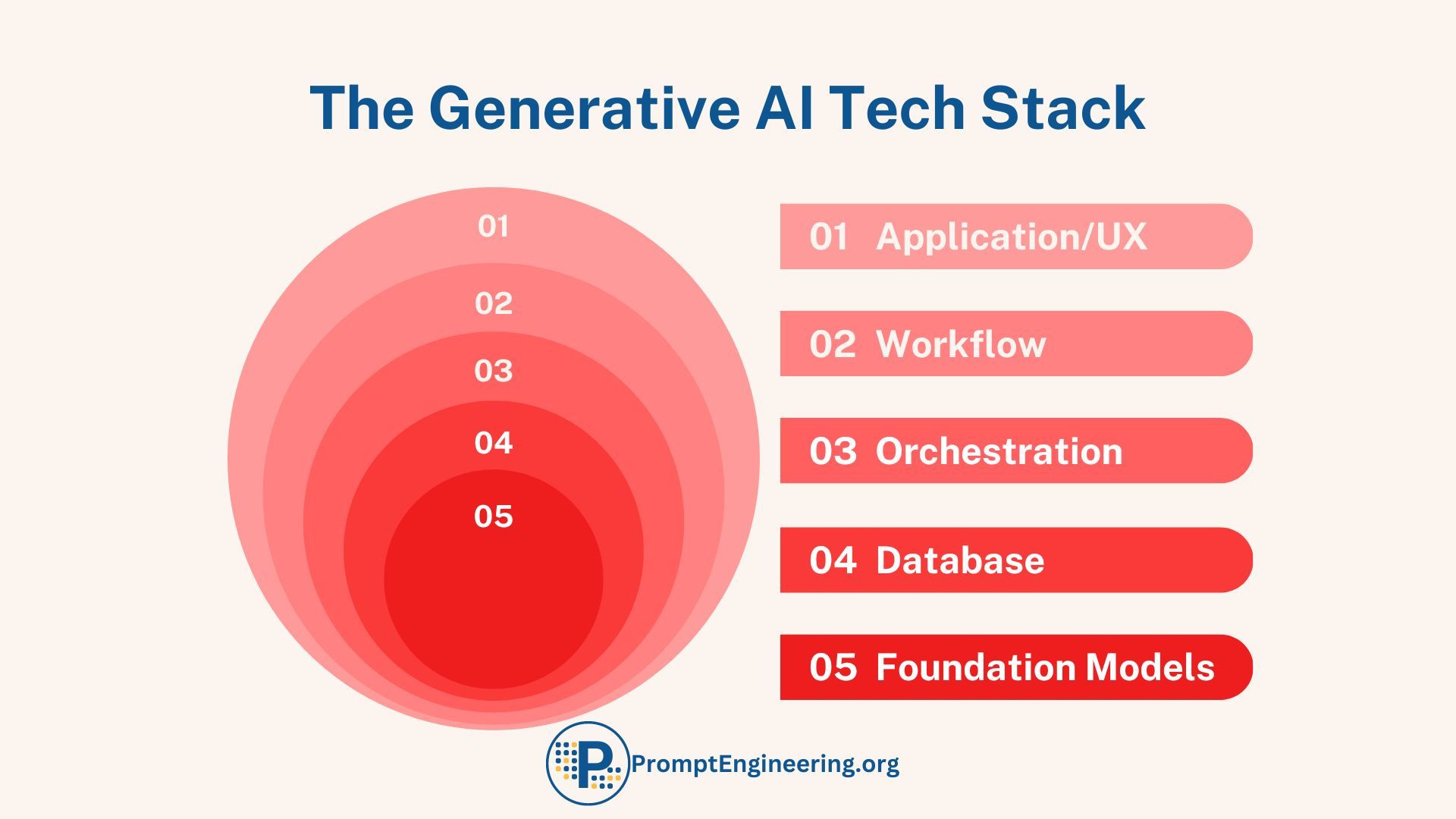
The guidelines cover critical actions across four key phases: 1) scoping a tight initial pilot, 2) laying the necessary foundations for success, 3) executing the focused pilot deployment, and 4) evaluating results and gradually expanding access. Within each phase, the article offers specific, tactical recommendations for elements like gathering requirements, training models, monitoring adoption, and addressing unintended consequences. Following this approach enables organizations to maximize value from AI while proactively mitigating risks and negative impacts.
Rather than reacting haphazardly to AI hype, companies need a rigorous implementation plan tailored to their environment. This guide equips executives, project teams, and change managers with expert advice and a proven playbook for launching AI successfully. The lessons and templates provided establish a responsible path to scaling technology that transforms operations without disrupting culture. With deliberate planning, AI can become an incredible force multiplier fueling enduring competitive advantage. This pragmatic guide charts the course.
Process Overview
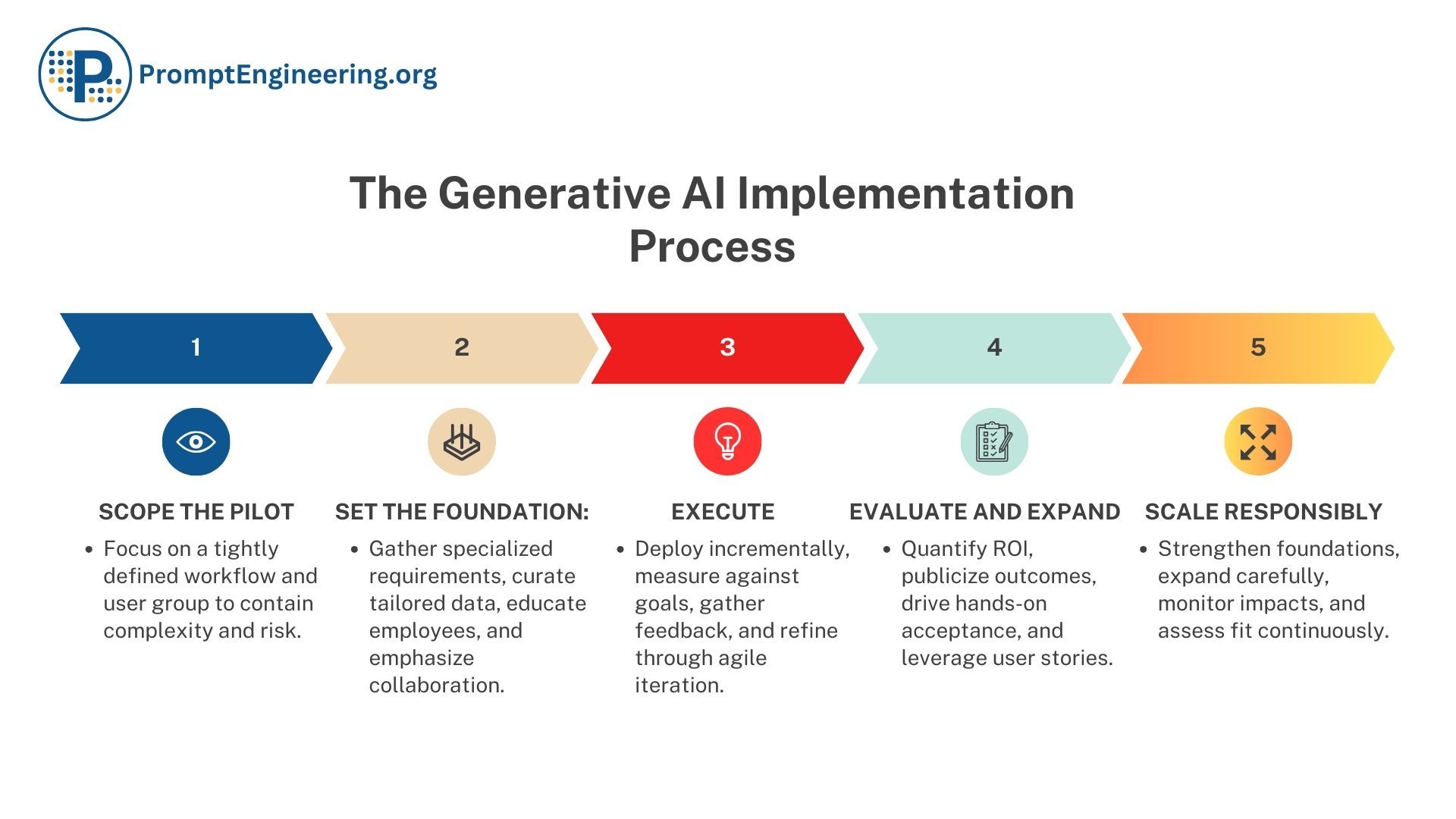
1. Scope the pilot:
- Focus on automating a narrowly defined workflow for a specific team
- Choose a well-understood process like monthly financial reporting
- Keep the initial scope limited to one team to contain complexity
2. Set the foundation:
- Gather requirements directly from the finance team
- Collect relevant data like past reports and inputs
- Educate employees extensively on AI, addressing concerns
- Emphasize AI augments work rather than replaces roles
- Refer to Strategic Framework for Enterprise Adoption of Generative AI and The Generative AI Stack
3. Execute the pilot:
- Start with a limited trial deployment to the finance team
- Measure success based on KPIs and gather user feedback
- Refine the solution through rapid iteration before expanding
4. Evaluate and expand:
- Quantify ROI through time/cost savings, productivity gains, etc.
- Publish pilot outcomes and user testimonials company-wide
- Use employee stories to proactively address concerns
- Allow small-scale exposure to drive broader adoption
5. Scale responsibly:
- Prioritize data, training, and prompts for the initial use case
- Only expand capabilities gradually once the foundation is solid
- Take a measured approach to responsible scaling
Scope the Pilot
When launching an AI implementation, the first phase is to scope a tightly defined pilot:
Focus on One Workflow
When determining the initial scope, resist the urge to automate a wide-ranging business process that spans multiple departments right away. Starting with a complex, cross-functional workflow introduces many variables and unknowns that increase the risk of failure.
Instead, target a singular, self-contained workflow within one team's domain. For example, automating the monthly financial reporting process for the finance department. This focused approach allows the pilot to concentrate on optimizing AI for a specific use case without convoluted dependencies.
Keeping the pilot confined to a discrete workflow within one team's control simplifies data requirements, training, and change management. It establishes clear success metrics based on the needs of known users. Beginning with a narrow scope creates a controlled environment for the pilot to demonstrate value. The organization can then take learnings and experience from this focused effort to inform expansion to additional workflows. However maintaining a limited initial focus gets the pilot grounded quickly with less complexity.
Pick a Structured Process
When selecting a workflow to automate for the initial pilot, choose an existing business process that is already well-defined and quantified by the organization. The ideal process will have established:
- Clear documentation of the required inputs, outputs, and steps
- Metrics already in place to measure performance and outcomes
- A rigorous understanding of how the process currently operates
- An owner team that is familiar with its nuances and pain points
For example, monthly financial reporting falls into this category for most finance departments. The data inputs, report components, review procedures, and metrics like speed and accuracy are typically standardized and understood.
Beginning with a structured process like financial reporting sets the pilot up for success. The known metrics and documentation make training the AI model simpler when clear goals are in place. A well-trodden process also simplifies change management when introducing automation into familiar territory. Starting with structured workflows maximizes the pilot's ability to enhance a quantified status quo.
Contain Scope
It is vital to keep the pilot tightly contained rather than attempt a full enterprise-wide rollout from the start. Limit participants to just the finance team directly involved in the targeted workflow.
Avoid extending access to other departments or allowing the pilot scope to balloon across multiple processes. Maintaining clear boundaries constrains the variables, data sources, and change impacts.
This concentrated approach allows the pilot to focus on optimizing AI specifically for the finance team's use case. If attempted at scale too soon, the solution may become diluted to a one-size-fits-none model that satisfies no one.
Starting small within a controlled environment enables tailoring to specialized users. It also reduces disruption and risk. The organization can then take learnings from the focused pilot to inform an incremental expansion strategy. But limiting initial scope is critical to manageable beginnings.
Tightly scoping the pilot concentrates impact while limiting variables. Defining a focused target workflow and user group establishes clear goals and simplifies initial data and integration needs. Containing the scope provides a controlled environment to demonstrate value before expanding.
Set the Foundation
With the pilot scoped, the next phase is laying its foundations for success:
Gather Specialized Requirements
Rather than make assumptions, directly engage the finance team to understand their unique goals and needs for the financial reporting workflow. Ask targeted questions to uncover:
- What are their desired outputs from an automated report? What insights add value?
- How do they measure success today in terms of report accuracy, speed, compliance, etc?
- What are their main pain points with the current manual process?
- What are their minimum requirements for adopting automation?
Recording these specialized requirements provides a clear target for training the AI model. It also establishes metrics aligned to the finance team's priorities to evaluate the pilot's success.
Tailoring requirements gathering to the pilot group and use case prevents misalignment with their real needs. The AI solution can then be optimized to solve the right problems for the right audience based on their direct input. Capturing their specialized requirements sets up targeted value.
Curate Relevant Data
With clear requirements from the finance team, the next foundational step is curating a dataset tailored to financial reporting:
- Collect examples of past source data used in financial reports, like revenue figures, cost projections, budgets, etc. This provides input examples.
- Gather a wide variety of prior financial reports to show different formatting styles, visualizations, narratives, and document structures. This provides target output examples.
- Inventory all the types of data sources, metrics, and report components that may be relevant. Identify any gaps.
- Work with the finance team to prioritize the most important and commonly used data and reports to focus on first.
This dedicated effort to assemble relevant training data accurately reflects the concepts and varieties involved in the finance team's reporting process. It provides the AI with targeted, quality examples to learn from. Curating a tailored dataset maximizes the applicability of the model to the actual workflow and environment.
Educate Employees Extensively
Before deployment, the organization should provide extensive training to all employees who will use or interact with the AI system:
- Offer general overviews explaining how AI and machine learning work at a basic level. Clarify common misperceptions.
- Provide in-depth technical training on interacting with generative models, prompt engineering, and interpreting outputs for teams directly involved in pilots.
- Spotlight case studies and examples demonstrating AI augmenting human work rather than replacing jobs.
- Directly address fears like downsizing and emphasize AI's role as an enhancer of human skills rather than human roles.
- Make training ongoing to keep pace with AI advancements and refresh knowledge.
Broad training establishes a baseline understanding across the organization, while role-specific programs skill up pilot teams to use the AI proficiently. Ongoing transparent education is crucial for securing buy-in and competency. Investing in developing an AI-literate workforce pays dividends.
Emphasize Collaboration
It is vital to position AI as a collaborative technology rather than an automated force:
- Frame AI as a productivity enhancer that takes over repetitive tasks to let human workers focus on higher judgement and creative responsibilities.
- Reinforce through messaging, training, and leadership communications that AI aims to augment human skills, not automate human roles.
- Demonstrate how AI will empower employees to be more innovative, find meaning, and advance their careers by working with technology.
- Proactively address fears of job loss by sharing plans to retrain and support any displaced workers.
Setting expectations that AI and human intelligence complement each other counters fears of automation. Change management should consistently reflect a vision of AI as a collaborator. This framing builds trust and acceptance. Responsible technology integrates people and machines.
Taking time to customize requirements and data to the use case maximizes relevance. Extensive education and emphasizing augmentation over automation secures crucial buy-in. This upfront foundation sets the pilot up to transform the target workflow for a receptive audience.
Execute the Pilot
With the foundations set, the next phase is executing a limited pilot deployment:
Deploy to the Chosen Team/Department
Once development reaches an initial viable stage, deployment should be limited to rolling out the AI solution only to the chosen finance team for a controlled trial period.
Tightly restricting access prevents exposure before the AI is ready while also limiting the impact radius. Only the target workflow owned by the finance team is automated during this trial phase.
Deploying incrementally allows gathering focused and actionable user feedback from the chosen team. Trying to support multiple processes and user types in early testing dilutes learnings. Concentrating the trial also allows refining the AI to optimally serve the finance team's specialized needs.
Maintaining tight deployment boundaries during initial testing is crucial. It enables tailoring and enhancing the solution for the target users without distraction before considering any broader release. Controlled exposure isolates impact until the organization is ready.
Measure Against Goals
Once deployed to the finance team, the pilot should be rigorously measured against the performance goals and success metrics defined during requirements gathering:
- Track quantitative metrics like reporting turnaround time, output accuracy, data processing speeds, and labor hours saved. Compare to existing benchmarks.
- Evaluate more qualitative goals like user satisfaction, workflow simplicity, and output usefulness using surveys and interviews.
- Review AI-generated outputs directly to validate compliance, formatting, and inclusion of desired insights.
- Assess against the minimum viable adoption criteria outlined by the finance team for performance, trust, and usefulness.
- Monitor system reliability, stability, and security.
Evaluating the pilot against predefined targets validates whether the AI solution is meeting the needs of the finance team and improving their reporting workflow. Regularly reviewing progress against these success criteria also informs required iterations and improvements to fully deliver on expectations before expanding further.
Gather User Feedback
In addition to performance data, extensive qualitative feedback should be gathered from pilot users through:
- Surveys asking about satisfaction, ease of use, trust, areas for improvement, and benefits seen.
- Interviews and small group discussions to understand detailed pain points and ideas.
- User observations and walkthroughs to identify usability issues and workflow hurdles.
- Focus groups to surface concerns, sentiments toward the technology, and feature requests.
- Open communication channels for users to provide feedback in the moment during use.
Regular user feedback channels provide crucial insights on adoption barriers, desired functionality, trust levels, and sentiment. Quantitative metrics demonstrate performance, but qualitative inputs reveal how real users are experiencing the technology. User-centered feedback guides refinement.
Refine Through Iteration
The insights uncovered from analyzing performance metrics and user feedback should drive rapid iteration of the AI solution while in the pilot stage.
Key refinement activities include:
- Addressing pain points and issues surfaced through user feedback. For example, improving explanations or interfaces.
- Incorporating new use cases or functionality requested by users to better suit their needs.
- Retraining models on expanded datasets to improve accuracy on core tasks.
- Optimizing prompts and workflows based on usability struggles observed.
- Fixing bugs and stability issues impacting reliability.
- Strengthening security reviewed during evaluations.
The goal is to quickly evolve the pilot AI capabilities in response to real-world learning until performance aligns with requirements and user adoption barriers are minimized. Refinement through agile iteration adapts the technology to the specialized environment.
Evaluate and Expand
Once the initial pilot demonstrates success, the next steps are evaluating results and strategically expanding:
Quantify ROI
A crucial step once the pilot concludes is quantifying the tangible ROI and business impacts achieved. Key performance data to analyze includes:
- Time and cost savings from process automation and efficiency gains
- Labour cost reductions through automated tasks requiring less employee time
- Revenue increases and profitability improvements enabled by the AI system
- Customer satisfaction scores based on metrics like report turnaround time
- Employee productivity metrics such as volume of reporting output
Compiling hard ROI metrics, cost-benefit analysis, and productivity data makes the concrete business case for expanding the AI solution more widely. The financial rationale convinces executives and stakeholders of the merits and urges adoption. Quantified outcomes turn anecdotal evidence into validated results.
Publicize Outcomes
To build momentum and alignment for scaling the AI solution, it is essential to publicize the pilot's success stories and results across the organization.
- Spotlight key performance metrics like reduced reporting time and cost savings through presentations, newsletters, intranet postings, etc.
- Create materials to educate others on how the AI system works and the benefits it delivers.
- Develop case studies and promotional media featuring video testimonials from finance team members highlighting ease of use, productivity increases, and improved work life.
- Have the CFO or executive sponsor publish an endorsement of the pilot results and their support for further AI adoption.
Widely sharing the positive outcomes and end-user experiences fosters organization-wide receptivity. Highlighting ROI data makes the value tangible while testimonials give it a relatable voice. Visible executive advocacy lends credibility. Savvy promotion seeds the appetite to uptake AI capabilities.
Drive Acceptance
Rather than open access at scale, the next phase is to gradually drive adoption by allowing hands-on interaction for new small groups:
- Identify adjacent departments with suitable workflows to pilot the AI solution, such as accounts payable, budgeting, or operations analytics.
- Deploy limited access to these additional teams for contained trial periods.
- Encourage peer knowledge sharing, allowing the original finance pilot team to train these new users.
- Gather feedback to improve usability for different groups while addressing concerns.
- Use new successes to further demonstrate benefits and lower adoption barriers.
Expanding exposure and capability in controlled increments sustains momentum while acclimating more of the workforce to AI collaboration. Positive hands-on experiences for small groups at a time encourage organic acceptance and capability growth. Gradual immersion is key.
Leverage Stories
Storytelling from original pilot users is an effective tool to nurture acceptance as AI expands to new areas:
- Incorporate pilot team member stories, quotes, and testimonials into training programs and change management communications.
- Have respected finance team members present to other departments how AI improved their roles to head off automation concerns.
- Create simple videos showing employees collaborating seamlessly with AI to demystify the experience for the uninitiated.
- Widely share a collection of testimonials detailing ease of adoption, usefulness, and benefits gained to inspire confidence.
Positive narratives from peers who have direct experience make the benefits relatable. Employees are more receptive to hearing from fellow colleagues than corporate communications. User storytelling gives AI a human voice and face to effectively convey its value.
Scale Responsibly
As adoption spreads, it is critical to scale AI capabilities strategically:
Optimize the Foundation
As adoption spreads, resist the urge to chase shiny new AI capabilities. Maintain focus on incrementally improving the core components underlying existing implementations:
- Expand datasets through additional examples relevant to established use cases. Diverse, quality data improves model robustness.
- Leverage new data to further tune model training and enhance accuracy on priority tasks. Solid training prevents regression.
- Refine prompts and workflows based on user feedback to optimize human-AI collaboration. Usability enables adoption.
- Fix reliability issues and build in redundancies. Rigorously test new model versions before release.
While expanding access, the fundamentals of reliable data, training, and prompts cannot be neglected. Consistent optimization of this foundation sustains performance and keeps AI delivering value as it scales.
Expand Gradually
When expanding AI adoption to new departments and workflows, take an incremental approach:
- Start with areas that have similar processes and needs to the initial finance reporting use case. Accounting and inventory workflows are closer replicates.
- Focus expansion on one new use case at a time, concentrating resources on optimizing integration and refining foundations before moving to another.
- Limit the availability of new solutions until existing teams and workflows have fully stabilized adoption. Don't push forward prematurely.
- Move carefully in allowing DIY access for departments to build their own solutions. Mature governance, support systems, and organizational readiness first.
- Closely monitor results and sentiment as usage grows. Pause expansion if capabilities are struggling to keep up.
By carefully selecting where to expand capabilities next and moving slowly one step at a time, organizations integrate AI deliberately versus recklessly. Gradual scaling allows developing solid expertise, governance, and infrastructure incrementally to support responsible growth.
Assess Continuous Fit
As adoption spreads, it is critical to continuously assess indicators of performance and user sentiment:
- Maintain clear success metrics for each use case to evaluate ongoing effectiveness and ROI. Don't let metrics languish after initial deployment.
- Actively monitor user feedback channels such as surveys and discussions to stay apprised of emerging issues or concerns.
- Convene regular working sessions with pilot teams to discuss progress, challenges, and enhancement opportunities.
- Study usage data for trends indicating changes in tool reliability, frequency of use, or user confusion.
- Watch for signs of lagging adoption, workaround behaviours, or dissatisfaction signalling a poor fit.
Regular checks on performance and experience identify areas needing improvement before small problems balloon. Continuously taking the pulse preserves fit between AI capabilities and evolving real-world needs. Proactive monitoring enables course correcting ahead of crises.
Consider Unintended Impacts
As AI becomes further embedded, organizations must study its broader influences:
- Assess impacts on factors like employee engagement, skills relevancy, job satisfaction, and organizational culture.
- Audit for biases creeping into data or algorithms that may discriminate against certain groups.
- Identify shifts in social dynamics, communication norms, and power structures resulting from AI collaboration.
- Survey customer attitudes toward increasing reliance on AI in products and services. Watch for backlash.
- Model second and third-order effects on markets, society, and the workforce as capabilities advance. Plan mitigations.
The Need for Dedicated AI Leadership
As AI promises to fundamentally reshape business models and supercharge operations, many leading organizations have recognized the need for dedicated leadership to fully harness its potential.
Appointing a Chief AI Officer (CAIO) provides this critical leadership to oversee and spearhead unified AI strategies and implementations across the organization.
AI is far more than the latest IT tool - it represents a genuinely transformative force for industries when strategically leveraged. The impacts of AI cut across functions, with the power to drive step-change improvements in everything from supply chain to HR.
Recognizing AI's extraordinary breadth, companies need a visionary CAIO leading a cross-functional approach. As capabilities permeate every process, the CAIO promotes widespread adoption for competitive differentiation, not just isolated use cases.
With rapid AI advancements requiring continuous learning, the CAIO drives the talent, infrastructure, and strategic vision to succeed. They bring much-needed leadership in navigating the algorithmic future.
As AI-driven transformation intensifies across industries, having a dedicated CAIO role will only grow more crucial for organizational success. The CAIO is essential for companies to fully harness the promise of AI.
Conclusion
Early detection of inadvertent consequences, both internal and external, allows redress before they become entrenched. Studying the broader effects of AI integration fosters responsible innovation aligned with ethical principles. Anticipating the system-wide impacts ensures AI scales sustainably.
Implementing transformative technologies like AI demands thoughtful orchestration and patient execution. Organizations cannot afford to scale capabilities recklessly and hope for the best. This guide outlines a rigorous four-phase approach with specific recommendations to integrate AI responsibly and sustainably.
Following these guidelines, companies can ensure pilots deliver targeted value, expand capabilities gradually, monitor for issues, and mitigate unintended consequences. The playbook covers critical actions from scoping projects tightly to evaluating incremental expansions and planning for second-order societal impacts. Executed well, AI implementations will transform workflows and enhance human potential rather than causing disruption.
With a pragmatic roadmap based on real-world lessons, business leaders can confidently harness AI to boost productivity, uncover insights, and delight customers. However, scaling its use responsibly requires assessing and addressing risks through each deliberate phase. Mission success depends on work and workforce augmentation, not automation. By internalizing these lessons, organizations can implement AI systematically to fuel enduring prosperity, innovation and growth. The future remains bright with strategic guidance illuminating the path forward.