The application of AI and technology in healthcare holds great promise but also raises understandable fears. One of the biggest concerns is around the potential for AI systems to generate misinformation or incorrect diagnoses and treatment plans.
The Risk of Hallucinating Information
Large language models like GPT-3 demonstrate an impressive ability to generate human-like text about any topic, while often sounding quite credible. However, these systems do not actually understand the content they generate. They hallucinate information, making up plausible-sounding statements without being constrained by facts or reality.
This becomes especially troubling in high-stakes domains like healthcare. An AI assistant could convincingly describe symptoms, analyze test results, and recommend treatments that are completely fabricated. Without safeguards in place, patients and providers could be misled into following harmful advice.
Dangers of an Irresponsible AI Doctor
Unlike human doctors who undertake years of medical training, AI has no innate domain knowledge or conception of ethics. AI systems act as high-tech parrots, mimicking patterns in data. They will dutifully generate whatever output maximizes some defined success metric, regardless of its accuracy or danger.
Putting full trust in an AI as a virtual doctor is reckless given current limitations. Unlike a responsible human physician, AI bears no liability for the recommendations it provides. Yet a convincing AI assistant could persuade patients to follow inappropriate treatments. More transparency and accountability measures are needed.
Mitigating Hallucinations Through Retrieval Augmented Generation (RAG)
While the risks are real, techniques exist to reduce hallucinated information from AI systems. One promising approach is retrieval augmented generation.
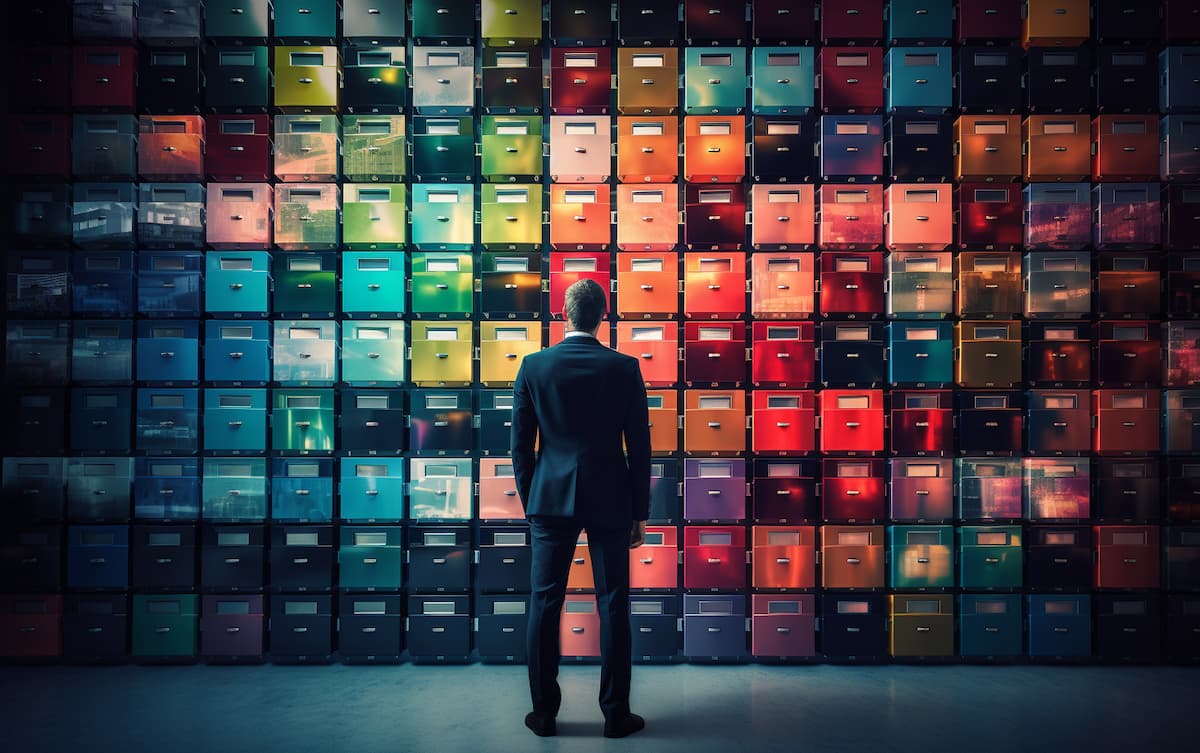
Incorporating Domain Knowledge
Large language models may have an impressive breadth of knowledge from pre-training on vast datasets but lack depth in specific domains like healthcare. Connecting LLMs to external knowledge sources can ground their outputs in facts and domain expertise they intrinsically lack.
Retrieval-augmented systems query databases of high-quality domain documents at generation time. By conditioning outputs on reliable retrieved information, they reduce unmoored hallucinations.
Enabling Transparency Through Sources
Retrieval augmentation provides transparency by linking AI-generated text to source materials. Users can verify the inputs supporting any outputs. For mission-critical applications like healthcare, it is not sufficient to blindly trust an AI system's assertions. The ability to validate against documents lends credibility.
Augmenting Existing Models
Retraining giant models like GPT-3 can be prohibitively expensive and compute-intensive. But retrieval augmentation can reduce hallucination for any existing LLM without costly modification, by simply supplementing it with a domain-relevant document database.
No single technique will eliminate risks from AI generation. However conditioning language models on quality external knowledge sources offers a promising path to reducing dangerous hallucinations.
Moving Forward Responsibly
The potential for AI and generative models to transform healthcare is exciting but also raises understandable concerns around misinformation and patient harm. Unconstrained language models hallucinate dangerously unreliable outputs. However, techniques like retrieval augmented generation offer a path to grounding AI reasoning in facts and domain knowledge.
There are no perfect solutions that eliminate risks entirely. But through responsible research and transparency measures that validate AI outputs against source data, we can work to ensure these technologies are developed safely.
If deployed deliberately, AI generation can augment human expertise, surface insights from vast medical literature, and expand healthcare access. But we must prioritize safety and accountability in our eagerness to innovate. With care, diligence, and scrutiny of these emerging systems, we can unlock their benefits while safeguarding patients. The prudent way forward is to embrace the opportunity of AI in healthcare, but resist the lure of quickly giving it unchecked authority.